What the Microsoft Outage Reveals
The surprising reason our technological systems keep failing
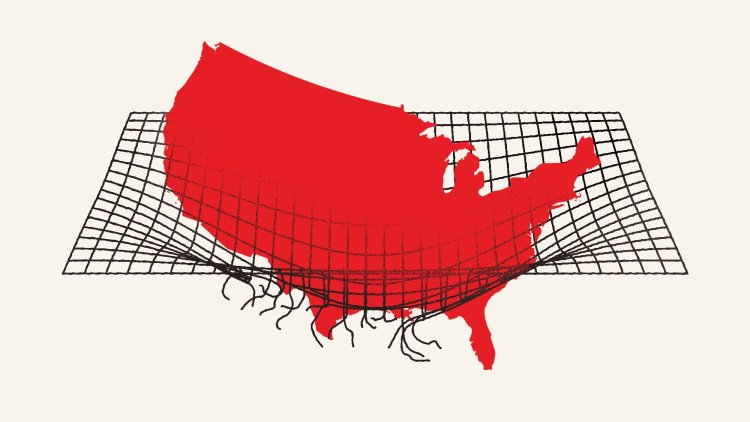
It happened again: yet another cascading failure of technology. In recent years we’ve had internet blackouts, aviation-system debacles, and now a widespread outage due to an issue affecting Microsoft systems, which has grounded flights and disrupted a range of other businesses, including health-care providers, banks, and broadcasters.
Why are we so bad at preventing these? Fundamentally, because our technological systems are too complicated for anyone to fully understand. These are not computer programs built by a single individual; they are the work of many hands over the span of many years. They are the interaction of countless components that might have been designed in a specific way for reasons that no one remembers. Many of our systems involve massive numbers of computers, any one of which might malfunction and bring down all the rest. And many have millions of lines of computer code that no one entirely grasps.
We don’t appreciate any of this until things go wrong. We discover the fragility of our technological infrastructure only when it’s too late.
So how can we make our systems fail less often?
We need to get to know them better. The best way to do this, ironically, is to break them. Much as biologists irradiate bacteria to cause mutations that show us how the bacteria function, we can introduce errors into technologies to understand how they’re liable to fail.
[Samuel Arbesman: Everything is overcomplicated]
This work often falls to software-quality-assurance engineers, who test systems by throwing lots of different inputs at them. A popular programming joke illustrates the basic idea.
A software engineer walks into a bar. He orders a beer. Orders zero beers. Orders 99,999,999,999 beers. Orders a lizard. Orders -1 beers. Orders a ueicbksjdhd. (So far, so good. The bartender may not have been able to procure a lizard, but the bar is still standing.) A real customer walks in and asks where the bathroom is. The bar bursts into flames.
Engineers can induce only so many errors. When something happens that they didn’t anticipate, the network breaks down. So how can we expand the range of failures that systems are exposed to? As someone who studies complex systems, I have a few approaches.
One is called “fuzzing.” Fuzzing is sort of like that engineer at the bar, but on steroids. It involves feeding huge amounts of randomly generated input into a software program to see how the program responds. If it doesn’t fail, then we can be more confident that it will survive the real and unpredictable world. The first Apple Macintosh was bolstered by a similar approach.
Fuzzing works at the level of individual programs, but we also need to inject failure at the system level. This has become known as “chaos engineering.” As a manifesto on the practice points out, “Even when all of the individual services in a distributed system are functioning properly, the interactions between those services can cause unpredictable outcomes.” Combine unpredictable outcomes with disruptive real-world events and you get systems that are “inherently chaotic.” Manufacturing that chaos in the engineering phase is crucial for reducing it in the wild.
Netflix was an early practitioner of this. In 2012, it publicly released a software suite it had been using internally called Chaos Monkey that randomly took down different subsystems to test how the company’s overall infrastructure would respond. This helped Netflix anticipate and guard against systemic failures that fuzzing couldn’t have caught.
[Read: The coming software apocalypse]
That being said, fuzzing and chaos engineering aren’t perfect. As our technological systems grow more complex, testing every input or condition becomes impossible. Randomness can help us find additional errors, but we will only ever be sampling a tiny subset of potential situations. And those don’t include the kinds of failures that distort systems without fully breaking them. Those are disturbingly difficult to root out.
Contending with these realities requires some epistemological humility: There are limits to what we can know about how and when our technologies will fail. It also requires us to curb our impulse to blame system-wide failures on a specific person or group. Modern systems are in many cases simply too large to allow us to point to a single actor when something goes wrong.
In their book, Chaos Engineering, Casey Rosenthal and Nora Jones offer a few examples of system failures with no single culprit. One involves a large online retailer who, in an effort to avoid introducing bugs during the holiday season, temporarily stopped making changes to its software code. This meant the company also paused its frequent system resets, which those changes required. Their caution backfired. A minor bug in an external library that the retailer used began to cause memory issues—problems that frequent resetting would have rendered harmless—and outages ensued.
In cases like this one, the fault is less likely to belong to any one engineer than to the inevitable complexity of modern software. Therefore, as Rosenthal and Jones argue—and as I explore in my book Overcomplicated—we must cope with that complexity by using techniques such as chaos engineering instead of trying to engineer it away.
As our world becomes more interconnected by massive systems, we need to be the ones breaking them—over and over again—before the world gets a chance.
What's Your Reaction?
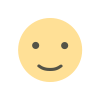
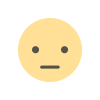
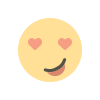
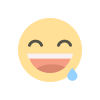
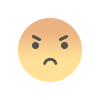
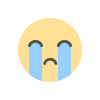
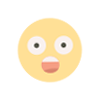