Ted Chiang Is Wrong About AI Art
It’s real. But it isn’t revolutionary.
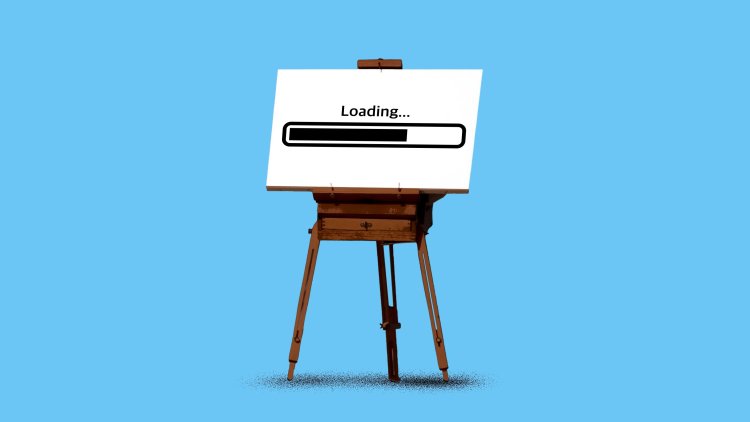
Artists and writers all over the world have spent the past two years engaged in an existential battle. Generative-AI programs such as ChatGPT and DALL-E are built on work stolen from humans, and machines threaten to replace the artists and writers who made the material in the first place. Their outrage is well warranted—but their arguments don’t always make sense or substantively help defend humanity.
Over the weekend, the legendary science-fiction writer Ted Chiang stepped into the fray, publishing an essay in The New Yorker arguing, as the headline says, that AI “isn’t going to make art.” Chiang writes not simply that AI’s outputs can be or are frequently lacking value but that AI cannot be used to make art, really ever, leaving no room for the many different ways someone might use the technology. Cameras, which automated realist painting, can be a tool for artists, Chiang says. But “a text-to-image generator? I think the answer is no.”
As in his previous writings on generative AI, here Chiang provides some sharp and necessary insights into an overwhelming societal shift. He correctly points out that the technology is predicated on a bias toward efficiency, and that these programs lack thought and intention. And I agree with Chiang that using AI to replace human minds for shareholder returns is depressing.
Yet the details of his story are off. Chiang presents strange and limiting frameworks for understanding both generative AI and art, eliminating important nuances in an ongoing conversation about what it means to be creative in 2024.
He makes two major mistakes in the essay, first by suggesting that what counts as “art” is primarily determined by the amount of effort that went into making it, and second that a program’s “intelligence” can or should be measured against an organic mind as opposed to being understood on its own terms. As a result, though he clearly intends otherwise, Chiang winds up asking his reader to accept a constrained view of human intelligence, artistic practice, and the potential of this technology—and perhaps even of the value of labor itself.
[Read: We’re witnessing the birth of a new artistic medium]
People will always debate the definition of art, but Chiang offers “a generalization: art is something that results from making a lot of choices.” A 10,000-word story, for instance, requires some 10,000 choices; a painting by Georges Seurat, composed of perhaps 220,000 dabs of paint, required 220,000 choices. By contrast, you make very few choices when prompting a generative-AI model, perhaps the “hundred choices” in a 100-word prompt; the program makes the rest for you, and because generative AI works by finding and mimicking statistical patterns in existing writing and images, he writes, those decisions are typically boring, too. Photographers, Chiang allows, make sufficient choices to be artists; users of AI, he predicts, never will.
What ratio of human decisions to canvas size or story length qualifies something as “art”? That glib question points to a more serious issue with Chiang’s line of thinking: You do not need to demonstrate hours of toil, make a lot of decisions, or even express thoughts and feelings to make art. Assuming that you do impoverishes human creativity.
Some of the most towering artists and artistic movements in recent history have divorced human skill and intention from their ultimate creations. Making a smaller number of decisions or exerting less intentional control does not necessarily imply less vision, creativity, brilliance, or meaning. In the early 1900s, the Dada and surrealist art movements experimented with automatism, randomness, and chance, such as in a famous collage made by dropping strips of paper and pasting them where they landed, ceding control to gravity and removing expression of human interiority; Salvador Dalí fired ink-filled bullets to randomly splatter lithographic stones. Decades later, abstract painters including Jackson Pollock, Joan Mitchell, and Mark Rothko marked their canvases with less apparent technical precision or attention to realism—seemingly random drips of pigment, sweeping brushstrokes, giant fields of color—and the Hungarian-born artist Vera Molnar used simple algorithms to determine the placement of lines, shapes, and colors on paper. Famed Renaissance artists used mathematical principles to guide their work; computer-assisted and algorithmic art today abounds. Andy Warhol employed mass production and called his studio the “Factory.” For decades, authors and artists such as Tristan Tzara, Samuel Beckett, John Cage, and Jackson Mac Low have used chance in their textual compositions.
Chiang allows that, under exceedingly rare circumstances, a human might work long and hard enough with a generative-AI model (perhaps entering “tens of thousands of words into its text box”) to “deserve to be called an artist.” But even setting aside more avant-garde or abstract applications, defining art primarily through “perspiration,” as Chiang does, is an old, consistently disproven tack. Édouard Manet, Claude Monet, and other associated 19th-century painters were once ridiculed by the French art establishment because their canvases weren’t as realistic as, and didn’t require the effort of, academic realism. “The newest version of DALL-E,” Chiang writes, “accepts prompts of up to four thousand characters—hundreds of words, but not enough to describe every detail of a scene.” Yet Manet’s and Monet’s Impressionist paintings—so maligned because the pictures involved fewer brushstrokes and thus fewer decisions, viewed through Chiang’s framework—shifted the trajectory of visual art and are today celebrated as masterpieces.
In all of these cases, humans devoted time and attention to conceiving each work—as artists using AI might as well. Although Chiang says otherwise, of course AI can be likened to a camera, or many other new technologies and creative mediums that attracted great ire when they were first introduced—radio, television, even the novel. The modern notion of automation via computing that AI embodies was partially inspired by a technology with tremendous artistic capacity: the Jacquard loom, a machine that weaves complex textiles based on punch-card instructions, just like the zeroes and ones of binary code. The Jacquard loom, itself a form of labor automation, was also in some sense a computer that humans could use to make art. Nobody would seriously argue that this means that many Bauhaus textiles and designs—foundational creative influences—are not art.
I am not arguing that a romance novel or still life created by a generative-AI model would inherently constitute art, and I’ve written previously that although AI products can be powerful tools for human artists, they are not quasi-artists themselves. But there isn’t a binary between asking a model for a complete output and sweating long hours before a blank page or canvas. AI could help iterate at many stages of the creative process: role-playing a character or visualizing color schemes or, in its “hallucinations,” offering a creative starting point. How a model connects words, images, and knowledge bases across space and time could be the subject of art, even a medium in itself. AI need not make art ex nihilo to be used to make artworks, sometimes fascinating ones; examples of people using the technology this way already abound.
[Read: The future of writing is a lot like hip-hop]
The impetus to categorically reject AI’s creative potential follows from Chiang’s other major misstep—the common but flawed criticism that AI programs, because they can’t adapt to novel situations as humans and animals do, are not truly “intelligent.” Chiang makes a comparison between rats and AlphaZero, a famous AI that effectively trained itself to play chess well: In an experimental setting, the rodents developed a new skill in 24 trials, and AlphaZero took 44 million trials to master chess. Ergo, he concludes, rats are intelligent and AlphaZero is not.
Yet dismissing the technology as little more than “auto-complete,” as Chiang does multiple times in his essay, is a category error. Of course an algorithm won’t capture our minds’ and bodies’ expressive intent and subjectivity—one is built from silicon, zeroes, and ones; the others, from organic elements and hundreds of millions of years of evolution. It should be as obvious that AI models, in turn, can do all sorts of things our brains can’t.
That distinction is an exciting, not damning, feature of generative AI. These computer programs have unfathomably more computing power and time available; rats and humans have finite brain cells and only a short time on Earth. As a result, the sorts of problems AI can solve, and how, are totally different. There are surely patterns and statistical relationships among the entirety of digitized human writing and visual art a machine can find that a person would not, at least not without several lifetimes. In this stretch of the essay, Chiang is citing an approach to measuring intelligence that comes from the computer scientist François Chollet. Yet he fails to acknowledge that Chollet, while seeking a way to benchmark AI programs against humans, also noted in the relevant paper that “many possible definitions of intelligence may be valid, across many different contexts.”
Another problem with arguing that some high number of decisions is what makes something art is that, in addition to being inaccurate, it risks implying that less intentional, “heartfelt,” or decision-rich jobs and tasks aren’t as deserving of protection. Chiang extends his point about effort to nonartistic and “low-quality text” as well: An email or business report warrants attention only “when the writer put some thought into it.” But just as making fewer choices doesn’t inherently mean someone doesn’t “deserve” to be deemed an artist, just because somebody completes rote tasks at work or writes a report on a deadline doesn’t mean that the output is worthless or that a person losing their job to an AI product is reasonable.
There are all sorts of reasons to criticize generative AI—the technology’s environmental footprint, gross biases, job displacement, easy creation of misinformation and nonconsensual sexual images, to name a few—but Chiang is arguing on purely creative and aesthetic grounds. Although he isn’t valuing some types of work or occupations over others, his logic leads there: Staking a defense of human labor and outputs, and human ownership of that labor and those outputs, on AI being “just” vapid statistics implies the jobs AI does replace might also be “just” vapid statistics. Defending human labor from AI should not be conflated with adjudicating the technology’s artistic merit. The Jacquard loom, despite its use as a creative tool, was invented to speed up and automate skilled weaving. The widespread job displacement and economic upheaval it caused mattered regardless of whether it was replacing or augmenting artistic, artisanal, or industrial work.
Chiang’s essay, in a sense, frames art not just as a final object but also as a process. “The fact that you’re the one who is saying it,” he writes, “the fact that it derives from your unique life experience and arrives at a particular moment in the life of whoever is seeing your work, is what makes [art] new.” I agree, and would go a step further: The processes through which art arises are not limited and cannot be delimited by a single artist or viewer but involve societies and industries and, yes, technologies. Surely, humans are creative enough to make and even desire a space for generative AI in that.
What's Your Reaction?
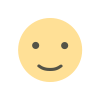
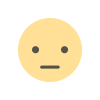
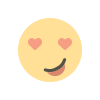
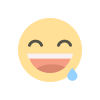
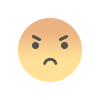
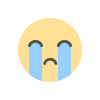
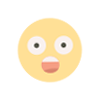