How to Keep Watch
Capturing reality is harder than it seems.

With smartphones in our pockets and doorbell cameras cheaply available, our relationship with video as a form of proof is evolving. We often say “pics or it didn’t happen!”—but meanwhile, there’s been a rise in problematic imaging including deepfakes and surveillance systems, which often reinforce embedded gender and racial biases. So what is really being revealed with increased documentation of our lives? And what’s lost when privacy is diminished?
In this episode of How to Know What’s Real, staff writer Megan Garber speaks with Deborah Raji, a Mozilla fellow, whose work is focused on algorithmic auditing and evaluation. In the past, Raji worked closely with the Algorithmic Justice League initiative to highlight bias in deployed AI products.
Listen to the episode here:
Listen and subscribe here: Apple Podcasts | Spotify | YouTube | Pocket Casts
The following is a transcript of the episode:
Andrea Valdez: You know, I grew up as a Catholic, and I remember the guardian angel was a thing that I really loved that concept when I was a kid. But then when I got to be, I don’t know, maybe around seven or eight, like, your guardian angel is always watching you. At first it was a comfort, and then it turned into kind of like a: Are they watching me if I pick my nose? Do they watch me?
Megan Garber: And are they watching out for me, or are they just watching me?
Valdez: Exactly. Like, are they my guardian angel or my surveillance angel? Surveillance angel.
Valdez: I’m Andrea Valdez. I’m an editor at The Atlantic.
Garber: And I’m Megan Garber, a writer at The Atlantic. And this is How to Know What’s Real.
Garber: I just got the most embarrassing little alert from my watch. And it’s telling me that it is, quote, “time to stand.”
Valdez: Why does it never tell us that it’s time to lie down?
Garber: Right. Or time to just, like, go to the beach or something? And it’s weird, though, because I’m realizing I’m having these intensely conflicting emotions about it. Because in one way, I appreciate the reminder. I have been sitting too long; I should probably stand up. But I don’t also love the feeling of just sort of being casually judged by a piece of technology.
Valdez: No, I understand. I get those alerts, too. I know it very well. And you know, it tells you, “Stand up; move for a minute. You can do it.” Uh, you know, you can almost hear it going, like, “Bless your heart.”
Garber: “Bless your lazy little heart.” The funny thing, too, about it is, like, I find myself being annoyed, but then I also fully recognize that I don’t really have a right to be annoyed, because I’ve asked them to do the judging.
Valdez: Yes, definitely. I totally understand. I mean, I’m very obsessed with the data my smartwatch produces: my steps, my sleeping habits, my heart rate. You know, just everything about it. I’m just obsessed with it. And it makes me think—well, I mean, have you ever heard of the quantified-self movement?
Garber: Oh, yeah.
Valdez: Yeah, so quantified self. It’s a term that was coined by Wired magazine editors around 2007. And the idea was, it was this movement that aspired to be, quote, unquote, “self-knowledge through numbers.” And I mean, it’s worth remembering what was going on in 2007, 2008. You know, I know it doesn’t sound that long ago, but wearable tech was really in its infancy. And in a really short amount of time, we’ve gone from, you know, Our Fitbit to, as you said, Megan, this device that not only scolds you for not standing up every hour—but it tracks your calories, the decibels of your environment. You can even take an EKG with it. And, you know, when I have my smartwatch on, I’m constantly on guard to myself. Did I walk enough? Did I stand enough? Did I sleep enough? And I suppose it’s a little bit of accountability, and that’s nice, but in the extreme, it can feel like I’ve sort of opted into self-surveillance.
Garber: Yes, and I love that idea in part because we typically think about surveillance from the opposite end, right? Something that’s done to us, rather than something that we do to ourselves and for ourselves. Watches are just one example here, right? There’s also smartphones, and there’s this broader technological environment, and all of that. That whole ecosystem, it all kind of asks this question of “Who’s really being watched? And then also, who’s really doing the watching?”
Valdez: Mm hmm. So I spoke with Deb Raji, who is a computer scientist and a fellow at the Mozilla Foundation. And she’s an expert on questions about the human side of surveillance, and thinks a lot about how being watched affects our reality.
—
Garber: I’d love to start with the broad state of surveillance in the United States. What does the infrastructure of surveillance look like right now?
Deborah Raji: Yeah. I think a lot of people see surveillance as a very sort of “out there in the world,” physical-infrastructure thing—where they see themselves walking down the street, and they notice a camera, and they’re like, Yeah, I’m being surveilled. Um, which does happen if you live in New York, especially post-9/11: like, you are definitely physically surveilled. There’s a lot of physical-surveillance infrastructure, a lot of cameras out there. But there’s also a lot of other tools for surveillance that I think people are less aware of.
Garber: Like Ring cameras and those types of devices?
Raji: I think when people install their Ring product, they’re thinking about themselves. They’re like, Oh, I have security concerns. I want to just have something to be able to just, like, check who’s on my porch or not. And they don’t see it as surveillance apparatus, but it ends up becoming part of a broader network of surveillance. And then I think the one that people very rarely think of—and again, is another thing that I would not have thought of if I wasn’t engaged in some of this work—is online surveillance. Faces are sort of the only biometric; uh, I guess, you know, it’s not like a fingerprint. Like, we don’t upload our fingerprints to our social media. We’re very sensitive about, like, Oh, you know, this seems like important biometric data that we should keep guarded. But for faces, it can be passively collected and passively distributed without you having any awareness of it. But also, we’re very casual about our faces. So we upload it very freely onto the internet. And so, you know, immigration officers—ICE, for example—have a lot of online-surveillance tools, where they’ll monitor people’s Facebook pages, and they’ll use sort of facial recognition and other products to identify and connect online identities, you know, across various social-media platforms, for example.
Garber: So you have people doing this incredibly common thing, right? Just sharing pieces of their lives on social media. And then you have immigration officials treating that as actionable data. Can you tell me more about facial recognition in particular?
Raji: So one of the first models I actually built was a facial-recognition project. And so I’m a Black woman, and I noticed right away that there were not a lot of faces that look like mine. And I remember trying to have a conversation with folks at the company at the time. And it was a very strange time to be trying to have this conversation. This was like 2017. There was a little bit of that happening in the sort of natural-language processing space. Like, people were noticing, you know, stereotyped language coming out of some of these models, but no one was really talking about it in the image space as much—that, oh, some of these models don’t work as well for darker-skinned individuals or other demographics. We audited a bunch of these products that were these facial-analysis products, and we realized that these systems weren’t working very well for those minority populations. But also definitely not working for the intersection of those groups. So like: darker skin, female faces.
Garber: Wow.
Raji: Some of the ways in which these systems were being pitched at the time, were sort of selling these products and pitching it to immigration officers to use to identify suspects.
Gaber: Wow.
Raji: And, you know, imagine something that’s not 70 percent accurate, and it’s being used to decide, you know, if this person aligns with a suspect for deportation. Like, that’s so serious.
Garber: Right.
Raji: You know, since we’ve published that work, we had just this—it was this huge moment. In terms of: It really shifted the thinking in policy circles, advocacy circles, even commercial spaces around how well those systems worked. Because all the information we had about how well these systems worked, so far, was on data sets that were disproportionately composed of lighter-skin men. Right. And so people had this belief that, Oh, these systems work so well, like 99 percent accuracy. Like, they’re incredible. And then our work kind of showed, well, 99 percent accuracy on lighter-skin men.
Garber: And could you talk a bit about where tech companies are getting the data from to train their models?
Raji: So much of the data required to build these AI systems are collected through surveillance. And this is not hyperbole, right? Like, the facial-recognition systems, you know, millions and millions of faces. And these databases of millions and millions of faces that are collected, you know, through the internet, or collected through identification databases, or through, you know, physical- or digital-surveillance apparatus. Because of the way that the models are trained and developed, it requires a lot of data to get to a meaningful model. And so a lot of these systems are just very data hungry, and it’s a really valuable asset.
Garber: And how are they able to use that asset? What are the specific privacy implications about collecting all that data?
Raji: Privacy is one of those things that we just don’t—we haven’t been able to get to federal-level privacy regulation in the States. There’s been a couple states that have taken initiative. So California has the California Privacy Act. Illinois has a BIPA, which is sort of a Biometric Information Privacy Act. So that’s specifically about, you know, biometric data like faces. In fact, they had a really—I think BIPA’s biggest enforcement was against Facebook and Facebook’s collection of faces, which does count as biometric data. So in Illinois, they had to pay a bunch of Facebook users a certain settlement amount. Yeah. So, you know, there are privacy laws, but it’s very state-based, and it takes a lot of initiative for the different states to enforce some of these things, versus having some kind of comprehensive national approach to privacy. That’s why enforcement or setting these rules is so difficult. I think something that’s been interesting is that some of the agencies have sort of stepped up to play a role in terms of thinking through privacy. So the Federal Trade Commission, FTC, has done these privacy audits historically on some of the big tech companies. They’ve done this for quite a few AI products as well—sort of investigating the privacy violations of some of them. So I think that that’s something that, you know, some of the agencies are excited about and interested in. And that might be a place where we see movement, but ideally we have some kind of law.
Garber: And we’ve been in this moment—this, I guess, very long moment—where companies have been taking the “ask for forgiveness instead of permission” approach to all this. You know, so erring on the side of just collecting as much data about their users as they possibly can, while they can. And I wonder what the effects of that will be in terms of our broader informational environment.
Raji: The way surveillance and privacy works is that it’s not just about the information that’s collected about you; it’s, like, your entire network is now, you know, caught in this web, and it’s just building pictures of entire ecosystems of information. And so, I think people don’t always get that. But yeah; it’s a huge part of what defines surveillance.
__
Valdez: Do you remember Surveillance Cameraman, Megan?
Garber: Ooh. No. But now I’m regretting that I don’t.
Valdez: Well, I mean, I’m not sure how well it was known, but it was maybe 10 or so years ago. There was this guy who had a camera, and he would take the camera and he would go and he’d stop and put the camera in people’s faces. And they would get really upset. And they would ask him, “Why are you filming me?” And, you know, they would get more and more irritated, and it would escalate. I think the meta-point that Surveillance Cameraman was trying to make was “You know, we’re surveilled all the time—so why is it any different if someone comes and puts a camera in your face when there’s cameras all around you, filming you all the time?”
Garber: Right. That’s such a great question. And yeah, the sort of difference there between the active act of being filmed and then the sort of passive state of surveillance is so interesting there.
Valdez: Yeah. And you know, that’s interesting that you say active versus passive. You know, it reminds me of the notion of the panopticon, which I think is a word that people hear a lot these days, but it’s worth remembering that the panopticon is an old idea. So it started around the late 1700s with the philosopher named Jeremy Bentham. And Bentham, he outlined this architectural idea, and it was originally conceptualized for prisons. You know, the idea was that you have this circular building, and the prisoners live in cells along the perimeter of the building. And then there’s this inner circle, and the guards are in that inner circle, and they can see the prisoners. But the prisoners can’t see the guards. And so the effect that Bantham was hoping this would achieve is that the prisoners would never know if they’re being watched—so they’d always behave as if they were being watched.
Garber: Mm. And that makes me think of the more modern idea of the watching-eyes effect. This notion that simply the presence of eyes might affect people’s behavior. And specifically, images of eyes. Simply that awareness of being watched does seem to affect people’s behavior.
Valdez: Oh, interesting.
Garber: You know, beneficial behavior, like collectively good behavior. You know, sort of keeping people in line in that very Bentham-like way.
Valdez: We have all of these, you know, eyes watching us now—I mean, even in our neighborhoods and, you know, at our apartment buildings. In the form of, say, Rng cameras or other, you know, cameras that are attached to our front doors. Just how we’ve really opted into being surveilled in all of the most mundane places. I think the question I have is: Where is all of that information going?
Garber: And in some sense, that’s the question, right? And Deb Raji has what I found to be a really useful answer to that question of where our information is actually going, because it involves thinking of surveillance not just as an act, but also as a product.
—
Raji: For a long time when you—I don’t know if you remember those, you know, “complete the picture” apps, or, like, “spice up my picture.” They would use generative models. You would kind of give them a prompt, which would be, like—your face. And then it would modify the image to make it more professional, or make it better lit. Like, sometimes you’ll get content that was just, you know, sexualizing and inappropriate. And so that happens in a nonmalicious case. Like, people will try to just generate images for benign reasons. And if they choose the wrong demographic, or they frame things in the wrong way, for example, they’ll just get images that are denigrating in a way that feels inappropriate. And so I feel like there’s that way in which AI for images has sort of led to just, like, a proliferation of problematic content.
Garber: So not only are those images being generated because the systems are flawed themselves, but then you also have people using those flawed systems to generate malicious content on purpose, right?
Raji: One that we’ve seen a lot is sort of this deepfake porn of young people, which has been so disappointing to me. Just, you know, young boys deciding to do that to young girls in their class; it really is a horrifying form of sexual abuse. I think, like, when it happened to Taylor Swift—I don’t know if you remember; someone used the Microsoft model, and, you know, generated some nonconsensual sexual images of Taylor Swift—I think it turned that into a national conversation. But months before that, there had been a lot of reporting of this happening in high schools. Anonymous young girls dealing with that, which is just another layer of trauma, because you’re like—you’re not Taylor Swift, right? So people don’t pay attention in the same way. So I think that that problem has actually been a huge issue for a very long time.
—
Garber: Andrea, I’m thinking of that old line about how if you’re not paying for something in the tech world, there’s a good chance you are probably the product being sold, right? But I’m realizing how outmoded that idea probably is at this point. Because even when we pay for these things, we’re still the products. And specifically, our data are the products being sold. So even with things like deepfakes—which are typically defined as, you know, using some kind of machine learning or AI to create a piece of manipulated media—even they rely on surveillance in some sense. And so you have this irony where these recordings of reality are now also being used to distort reality.
Valdez: You know, it makes me think of Don Fallis: this philosopher who talked about the epistemic threat of deepfakes and that it’s part of this pending infopocalypse. Which sounds quite grim, I know. But I think the point that Fallis was trying to make is that with the proliferation of deepfakes, we’re beginning to maybe distrust what it is that we’re seeing. And we talked about this in the last episode. You know, “seeing is believing” might not be enough. And I think we’re really worried about deepfakes, but I’m also concerned about this concept of cheap fakes, or shallow fakes. So cheap fakes or shallow fakes—it’s, you know, you can tweak or change images or videos or audio just a little bit. And it doesn’t actually require AI or advanced technology to create. So one of the more infamous instances of this was in 2019. Maybe you remember there was a video of Nancy Pelosi that came out where it sounded like she was slurring her words.
Garber: Oh, yeah, right. Yeah.
Valdez: Really, the video had just been slowed down using easy audio tools, and just slowed down enough to create that perception that she was slurring her words. So it’s a quote, unquote “cheap” way to create a small bit of chaos.
Garber: And then you combine that small bit of chaos with the very big chaos of deepfakes.
Valdez: Yeah. So one, the cheat fake is: It’s her real voice. It’s just slowed down—again, using, like, simple tools. But we’re also seeing instances of AI-generated technology that completely mimics other people’s voices, and it’s becoming really easy to use now. You know, there was this case recently that came out of Maryland where there was a high-school athletic director, and he was arrested after he allegedly used an AI voice simulation of the principal at his school. And he allegedly simulated the principal’s voice saying some really horrible things, and it caused all this blowback on the principal before investigators, you know, looked into it. Then they determined that the audio was fake. But again, it was just a regular person that was able to use this really advanced-seeming technology that was cheap, easy to use, and therefore easy to abuse.
Garber: Oh, yes. And I think it also goes to show how few sort of cultural safeguards we have in place right now, right? Like, the technology will let people do certain things. And we don’t always, I think, have a really well-agreed-upon sense of what constitutes abusing the technology. And you know, usually when a new technology comes along, people will sort of figure out what’s acceptable and, you know, what will bear some kind of safety net. Um, and will there be a taboo associated with it? But with all of these new technologies, we just don’t have that. And so people, I think, are pushing the bounds to see what they can get away with.
Valdez: And we’re starting to have that conversation right now about what those limits should look like. I mean, lots of people are working on ways to figure out how to watermark or authenticate things like audio and video and images.
Garber: Yeah. And I think that that idea of watermarking, too, can maybe also have a cultural implication. You know, like: If everyone knows that deepfakes can be tracked, and easily, that is itself a pretty good disincentive from creating them in the first place, at least with an intent to fool or do something malicious.
Valdez: Yeah. But. In the meantime, there’s just going to be a lot of these deepfakes and cheap fakes and shallow fakes that we’re just going to have to be on the lookout for.
—
Garber: Is there new advice that you have for trying to figure out whether something is fake?
Raji: If it doesn’t feel quite right, it probably isn’t. A lot of these AI images don’t have a good sense of, like, spatial awareness, because it’s just pixels in, pixels out. And so there’s some of these concepts that we as humans find really easy, but these models struggle with. I advise people to be aware of, like—sort of trust your intuition. If you’re noticing weird artifacts in the image, it probably isn’t real. I think another thing, as well, is who posts.
Garber: Oh, that’s a great one; yeah.
Raji: Like, I mute very liberally on Twitter; uh, any platform. I definitely mute a lot of accounts that I notice [are] caught posting something. Either like a community note or something will reveal that they’ve been posting fake images, or you just see it and you recognize the design of it. And so I just knew that kind of content. Don’t engage with those kind of content creators at all. And so I think that that’s also like another successful thing on the platform level. Deplatforming is really effective if someone has sort of three strikes in terms of producing a certain type of content. And that’s what happened with the Taylor Swift situation—where people were disseminating these, you know, Taylor Swift images and generating more images. And they just went after every single account that did that—you know, completely locked down her hashtag. Like, that kind of thing where they just really went after everything. Um, and I think that that’s something that we should just do in our personal engagement as well.
—
Garber: Andrea, that idea of personal engagement, I think, is such a tricky part of all of this. I’m even thinking back to what we were saying before—about Ring and the interplay we were getting at between the individual and the collective. In some ways, it’s the same tension that we’ve been thinking about with climate change and other really broad, really complicated problems. This, you know, connection between personal responsibility, but also the outsized role that corporate and government actors will have to play when it comes to finding solutions. Mm hmm. And with so many of these surveillance technologies, we’re the consumers, with all the agency that that would seem to entail. But at the same time, we’re also part of this broader ecosystem where we really don’t have as much control as I think we’d often like to believe. So our agency has this giant asterisk, and, you know, consumption itself in this networked environment is really no longer just an individual choice. It’s something that we do to each other, whether we mean to or not.
Valdez: Yeah; you know, that’s true. But I do still believe in conscious consumption so much as we can do it. Like, even if I’m just one person, it’s important to me to signal with my choices what I value. And in certain cases, I value opting out of being surveilled so much as I can control for it. You know, maybe I can’t opt out of facial recognition and facial surveillance, because that would require a lot of obfuscating my face—and, I mean, there’s not even any reason to believe that it would work. But there are some smaller things that I personally find important; like, I’m very careful about which apps I allow to have location sharing on me. You know, I go into my privacy settings quite often. I make sure that location sharing is something that I’m opting into on the app while I’m using it. I never let apps just follow me around all the time. You know, I think about what chat apps I’m using, if they have encryption; I do hygiene on my phone around what apps are actually on my phone, because they do collect a lot of data on you in the background. So if it’s an app that I’m not using, or I don’t feel familiar with, I delete it.
Garber: Oh, that’s really smart. And it’s such a helpful reminder, I think, of the power that we do have here. And a reminder of what the surveillance state actually looks like right now. It’s not some cinematic dystopia. Um, it’s—sure, the cameras on the street. But it’s also the watch on our wrist; it’s the phones in our pockets; it’s the laptops we use for work. And even more than that, it’s a series of decisions that governments and organizations are making every day on our behalf. And we can affect those decisions if we choose to, in part just by paying attention.
Valdez: Yeah, it’s that old adage: “Who watches the watcher?” And the answer is us.
__
Garber: That’s all for this episode of How to Know What’s Real. This episode was hosted by Andrea Valdez and me, Megan Garber. Our producer is Natalie Brennan. Our editors are Claudine Ebeid and Jocelyn Frank. Fact-check by Ena Alvarado. Our engineer is Rob Smierciak. Rob also composed some of the music for this show. The executive producer of audio is Claudine Ebeid, and the managing editor of audio is Andrea Valdez.
Valdez: Next time on How to Know What’s Real:
Thi Nguyen: And when you play the game multiple times, you shift through the roles, so you can experience the game from different angles. You can experience a conflict from completely different political angles and re-experience how it looks from each side, which I think is something like, this is what games are made for.
Garber: What we can learn about expansive thinking through play. We’ll be back with you on Monday.
What's Your Reaction?
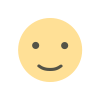
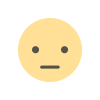
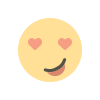
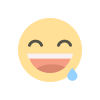
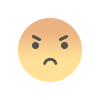
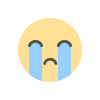
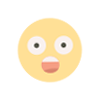