A Polygraph in Your Pocket
Can machine-learning algorithms distinguish truth from falsehood? We’re about to find out.
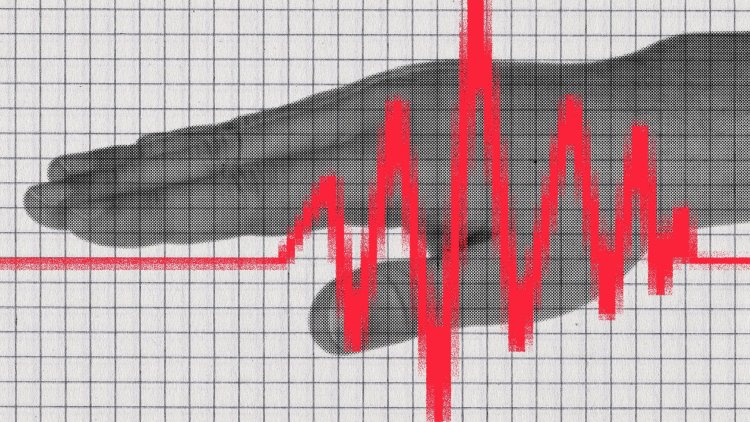
Journalists have a saying about the importance of confirming even the most basic facts: “If your mother says she loves you, check it out.” Recently, I decided to follow that advice literally, with the help of an AI-based lie detector.
The tool is called Coyote. Trained on a data set of transcripts in which people were established as having lied or told the truth, the machine-learning model then tells you whether a statement is deceptive. According to its creators, its textual analysis is accurate 80 percent of the time.
A few weeks ago, I called my mom. After some initial questioning to establish ground truth—how she spent her vacation in France, what she did that morning—I got to the point. “Do you love me?” I asked. She said yes. I asked why. She listed a handful of positive qualities, the kinds of things a son would be proud to hear—if they were true.
Later, I plugged a transcript of her answer into Coyote. The verdict: “Deception likely.”
People have been trying and failing to create a reliable lie detector for a very long time. The industry is never not booming; the polygraph accounts for $2 billion in business every year. Now a wave of newcomers is challenging the century-old device, catering to a ready market in the corporate world and law enforcement. The most cutting-edge of them claim to have cracked the case using artificial intelligence and machine learning, with accuracy levels purportedly as high as 93 percent.
Historically, every advance in the lie-detection field has failed to live up to the hype, and, indeed, these new tools seem to suffer from many of the same problems as older technologies, plus some new ones. But that probably won’t stop them from spreading. If the tech-world ethos of “Anything we can do, we will do” applies, we could soon have AI lie detectors lurking on our Zoom calls, programmed into our augmented-reality glasses, and downloaded onto our phones, analyzing everyday conversations in real time. In which case their unreliability might actually be a good thing.
Ask people how to spot a lie, and most will say the same thing: Liars avoid eye contact. This belief turns out to be false. Human beings think they’re good at detecting lies, but studies show that they’re only slightly more accurate than a coin flip.
The history of lie-detecting technology is one tool after another built on premises that are intuitive but wrong. The modern industry began in the early 20th century with the polygraph, which measured blood pressure, respiratory rate, and galvanic skin response (sweating), under the theory that guilty parties show greater arousal. Early critics pointed out that the polygraph detects anxiety, not dishonesty, and can be gamed. In 1988, Congress passed a law prohibiting companies from using lie detectors during hiring, and a 1998 Supreme Court ruling held that polygraph results can’t be used as evidence in federal court. Nonetheless, the FBI and CIA still use it, and it’s certainly effective at eliciting confessions from jittery subjects, guilty or not.
In the 1960s, the psychologist Paul Ekman theorized that body and facial movements can betray deception, a phenomenon he called “leakage.” Ekman’s work gave rise to a cottage industry of “body-language experts,” who could supposedly discern truth and falsehood from a speaker’s glances and fidgets. (It also inspired the TV series Lie to Me.) But Timothy R. Levine, a professor of communication studies at the University of Alabama at Birmingham, told me that the more researchers study deception cues, the smaller the effect size—which, he wrote in a blog post, makes these cues a “poster child” for the replication crisis in social sciences.
Language-based detection was the next frontier. Starting in the 1970s, studies found that liars use fewer self-references like I or we and more negative terms like hate or nervous. In the 1990s, researchers developed a system called reality monitoring, which is based on the theory that people recalling real memories will include more details and sensory information than people describing imagined events. A 2021 meta-analysis of 40 studies found that the reality-monitoring scores of truth tellers were meaningfully higher than those of liars, and in 2023, a group of researchers published an article in Nature arguing that the one reliable heuristic for detecting lies is level of detail.
Wall Street is a natural testing ground for these insights. Every quarter, executives present their best face to the world, and the investor’s job is to separate truth from puffery. Hedge funds have accordingly looked at language-based lie detection as a potential source of alpha.
In 2021, a former analyst named Jason Apollo Voss founded Deception and Truth Analysis, or DATA, with the goal of providing language-based lie detection to investors. Voss told me that DATA looks at 30 different language parameters, then clusters them into six categories, each based on a different theory of deception, including clarity (liars are vague), authenticity (liars are ingratiating), and tolerance (liars don’t like being questioned).
When I asked Voss for examples of DATA’s effectiveness, he pointed to Apple’s report for the third quarter of 2023, in which the company wrote that its “future gross margins can be impacted by a variety of factors … As a result, the Company believes, in general, gross margins will be subject to volatility and downward pressure.” DATA’s algorithm rated this statement as “strongly deceptive,” Voss said.
Three quarters later, Apple lowered its expectations about future gross margins. “So our assessment here was correct,” Voss said. But, I asked, where was the deception? They said their gross margins would be subject to downward pressure! Voss wrote in an email that the company’s lack of specificity amounted to “putting spin on the ball” rather than outright lying. “Apple is clearly obfuscating what the future results are likely to be,” he wrote.
Voss’s approach, for all its ostensible automation, still seemed fundamentally human: subjective, open to interpretation, and vulnerable to confirmation bias. Artificial intelligence, by contrast, offers the tantalizing promise of lie detection untainted by human intuition.
Until recently, every lie-detecting tool was based on a psychological thesis of deception: Liars sweat because they’re anxious; they avoid detail because they don’t have real memories to draw on. Machine-learning algorithms don’t need to understand. Show them enough pictures of dogs and they can learn to tell you whether something is a dog without really “knowing” what dog-ness means. Likewise, a model can theoretically be trained on reams of text (or audio or video recordings) labeled as deceptive or truthful and use the patterns it uncovers to detect lies in a new document. No psychology necessary.
Steven Hyde started researching language-based lie detection as a Ph.D. student in management at the University of Texas at San Antonio in 2015. He didn’t know how to code, so he recruited a fellow graduate student and engineer, Eric Bachura, and together they set out to build a lie detector to analyze the language of CEOs. “What if we could prevent the next Elizabeth Holmes?” Hyde recalls thinking. Part of the challenge was finding good training data. To label something a lie, you need to show not only that it was false, but also that the speaker knew it was false.
Hyde and Bachura looked for deception everywhere. They initially focused on corporate earnings calls in which statements were later shown to be false. Later, while building Coyote, Hyde added in speeches by politicians and celebrities. (Lance Armstrong was in there.) He also collected videos of deception-based game shows on YouTube.
A typical machine-learning tool would analyze the training data and use it to make judgments about new cases. But Hyde was wary of that brute-force approach, since it risked mislabeling something as truth or a lie because of confounding variables in the data set. (Maybe the liars in their set disproportionately talked about politics.) And so psychological theory crept back in. Hyde and Bachura decided to “teach” the algorithm how language-based lie detection works. First, they’d scan a piece of text for linguistic patterns associated with deception. Then they’d use a machine-learning algorithm to compare the statistical frequency of those elements in the document to the frequency of similar elements in the training data. Hyde calls this a “theory-informed” approach to AI.
When Hyde and Bachura tested their initial model, they found that it detected deception with 84 percent accuracy. “I was blown away,” Hyde said. “Like, no frickin’ way.” He used the tool to analyze Wells Fargo earnings calls from the period before the company was caught creating fake customer accounts. “Every time they talked about cross-sell ratio, it was coded as a lie,” he said—proof that the model was catching deceptive statements. (Hyde and Bachura later parted ways, and Bachura started a rival company called Arche AI.)
Hyde’s confidence made me curious to try out Coyote for myself. What dark truths would it reveal? Hyde’s business partner, Matthew Kane, sent over a link to the software, and I downloaded it onto my computer.
Coyote’s interface is simple: Upload a piece of text, audio, or video, then click “Analyze.” It then spits out a report that breaks the transcript into segments. Each segment gets a rating of “Truth likely” or “Deception likely,” plus a percentage score that represents the algorithm’s confidence level. (The scale essentially runs from negative 100, or totally dishonest, to positive 100, or totally truthful.) Hyde said there’s no official cutoff score at which a statement can be definitively called a lie, but suggested that for my purposes, any “Deception likely” score below 70 percent should be treated as true. (In my testing, I focused on text, because the audio and video software was buggy.)
I started out with the low-hanging fruit of lies. Bill Clinton’s 1998 statement to the grand jury investigating the Monica Lewinsky affair, in which he said that their encounters “did not constitute sexual relations,” was flagged as deceptive, but with a confidence level of just 19 percent—nowhere near Hyde’s suggested threshold score. Coyote was even less sure about O. J. Simpson’s statement in court asserting his innocence in 1995, labeling it deceptive with only 8 percent confidence. A wickedly treacherous soliloquy from Season 2 of my favorite reality show, The Traitors: 11 percent deceptive. So far, Coyote seemed to be a little gun-shy.
I tried lying myself. In test conversations with friends, I described fake vacation plans (spring break in Cabo), what I would eat for my last meal (dry gluten-free spaghetti), and my ideal romantic partner (cruel, selfish). To my surprise, over a couple of hours of testing, not a single statement rose above the 70 percent threshold that Hyde had suggested. Coyote didn’t seem to want to call a lie a lie.
[Read: Artificial intelligence is misreading human emotion]
What about true statements? I recruited friends to ask me questions about my life, and I responded honestly. The results were hard to make sense of. Talking about my morning routine: “Truth likely,” 2 percent confidence. An earnest speech about my best friend from middle school was coded as a lie, with 57 percent confidence. Telling my editor matter-of-factly about my reporting process for this story: 32 percent deception.
So according to Coyote, hardly any statements I submitted were obvious lies, nor were any clearly truthful. Instead, everything was in the murky middle. From what I could tell, there was no correlation between a statement’s score and its actual truth or falsehood. Which brings us back to my mom. When Coyote assessed her claim that she loved me, it reported that she was likely being deceptive—but its confidence level was only 14 percent. Hyde said that was well within the safe zone. “Your mom does love you,” he assured me.
I remained confused, though. I asked Hyde how it’s possible to claim that Coyote’s text analysis is 80 percent accurate if there’s no clear truth/lie cutoff. He said the threshold they used for accuracy testing was private.
Still, Coyote was a model of transparency compared to my experience with Deceptio.ai, a web-based lie detector. Despite the company’s name—and the fact that it bills itself as “AI-POWERED DECEPTION DETECTION”—the company’s CEO and co-founder, Mark Carson, told me in an email that he could not disclose whether his product uses artificial intelligence. That fact, he said, is “proprietary IP.” For my test-drive, I recorded myself making a truthful statement and uploaded the transcript. Among the suspicious terms that got flagged for being associated with deception: “actually” (could conceal undisclosed information), “afterwards” (indicates a passing of time in which you do not know what the subject was doing), and “but” (“stands for Behold the Underlying Truth”). My overall “truth score” was 68 percent, which qualified me as “deceptive.”
Deceptio.ai’s framework is based on the work of Mark McClish, who created a system called “Statement Analysis” while teaching interrogation techniques to U.S. marshals in the 1990s. When I asked McClish whether his system had a scientific foundation, he said, “The foundation is the English language.” I put the same question to Carson, Deceptio.ai’s founder. “This is a bit of ‘Trust me, bro’ science,” he said.
And maybe that’s enough for some users. A desktop app called LiarLiar purportedly uses AI to analyze facial movements, blood flow, and voice intonation in order to detect deception. Its founder, a Bulgarian engineer named Asen Levov, says he built the software in three weeks and released it last August. That first version was “very ugly,” Levov told me. Still, more than 800 users have paid between $30 and $100 to sign up for lifetime subscriptions, he said. He recently relaunched the product as PolygrAI, hoping to attract business clients. “I’ve never seen such early validation,” he said. “There’s so much demand for a solution like this.”
The entrepreneurs I spoke with all say the same thing about their lie detectors: They’re not perfect. Rather, they can help guide investigators by flagging possibly deceptive statements and inspiring further inquiry.
But plenty of businesses and law-enforcement agencies seem ready to put their faith in the tools’ judgments. In June, the San Francisco Chronicle revealed that police departments and prisons in California had used junk-science “voice-stress analysis” tests to assess job applicants and inmates. In one case, prison officials used it to discredit an inmate’s report of abuse by guards. Departments around the country subject 911 calls to pseudoscientific linguistic analysis to determine whether the callers are themselves guilty of the crimes they’re reporting. This has led to at least one wrongful murder conviction, ProPublica reported in December 2022. A 2023 federal class-action lawsuit in Massachusetts accused CVS of violating the state’s law against using lie detectors to screen job applicants after the company allegedly subjected interviewees to AI facial and vocal analysis. (CVS reached a tentative settlement with the lead plaintiff earlier this month.)
If the industry continues its AI-juiced expansion, we can expect a flood of false positives. Democratized lie detection means that prospective hires, mortgage applicants, first dates, and Olympic athletes, among others, would be falsely accused of lying all the time. This problem is unavoidable, Vera Wilde, a political theorist and scientist who studies research methodology, told me. There’s an “irresolvable tension,” she said, between the need to catch bad guys and creating so many false positives that you can’t sort through them.
And yet a future in which we’re constantly being subjected to faulty lie-detection software might be the best path available. The only thing scarier than an inaccurate lie detector would be an accurate one.
[Read: The lie detector in the age of alternative facts]
Lying is essential. It lubricates our daily interactions, sparing us from one another’s harshest opinions. It helps people work together even when they don’t agree and enables those with less power to protect themselves by blending in with the tribe. Exposing every lie would threaten the very concept of a self, because the version of ourselves we show the world is inherently selective. A world without lying would be a world without privacy.
Profit-driven companies have every incentive to create that world. Knowing a consumer’s true beliefs is the holy grail of market research. Law-enforcement personnel who saw Minority Report as an aspirational tale rather than a cautionary one would pay top dollar to learn what suspects are thinking. And who wouldn’t want to know whether their date was really into them? Devin Liddell, whose title is “principal futurist” at the design company Teague, says he could see lie-detection tools getting integrated into wearables and offering running commentary on our chatter, perhaps through a discreet earpiece. “It’s an extrasensory superpower,” Liddell told me.
Some companies are already exploring these options. Carson said Deceptio.ai is talking to a large dating platform about a partnership. Kane said he was approached by a Zoom rival about integrating Coyote. He expects automated language-based tools to overtake the polygraph, because they don’t require human administration.
I asked Hyde if he uses Coyote to analyze his own interactions. “Hell no,” he said. “I think it would be a bad thing if everyone had my algorithm on their phone, running it all the time. That would be a worse world.” Hyde said he wants to mitigate any damage the tool might inflict. He has avoided pitching Coyote to the insurance industry, a sector that he considers unethical, and he doesn’t want to release a retail version. He reminded me of the leaders of generative-AI companies who agonize publicly over the existential risk of superintelligent AI while insisting that they have no choice but to build it. “Even if Coyote doesn’t work out, I have zero doubt this industry will be successful,” Hyde said. “This technology will be in our lives.”
Hyde grew up Mormon, and when he was 19 the Church sent him on his mission to Peoria, Illinois. One day, one of the other missionaries came out to him. That man, Shane, is now one of Hyde’s best friends. Shane eventually left the Church, but for years he remained part of the community. Hyde thinks often about the number of times Shane must have lied to survive.
“The ability to deceive is a feature, not a bug,” Hyde said. No lies detected.
What's Your Reaction?
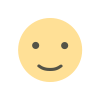
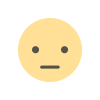
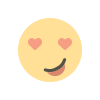
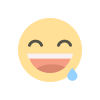
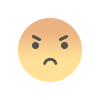
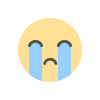
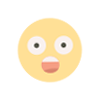